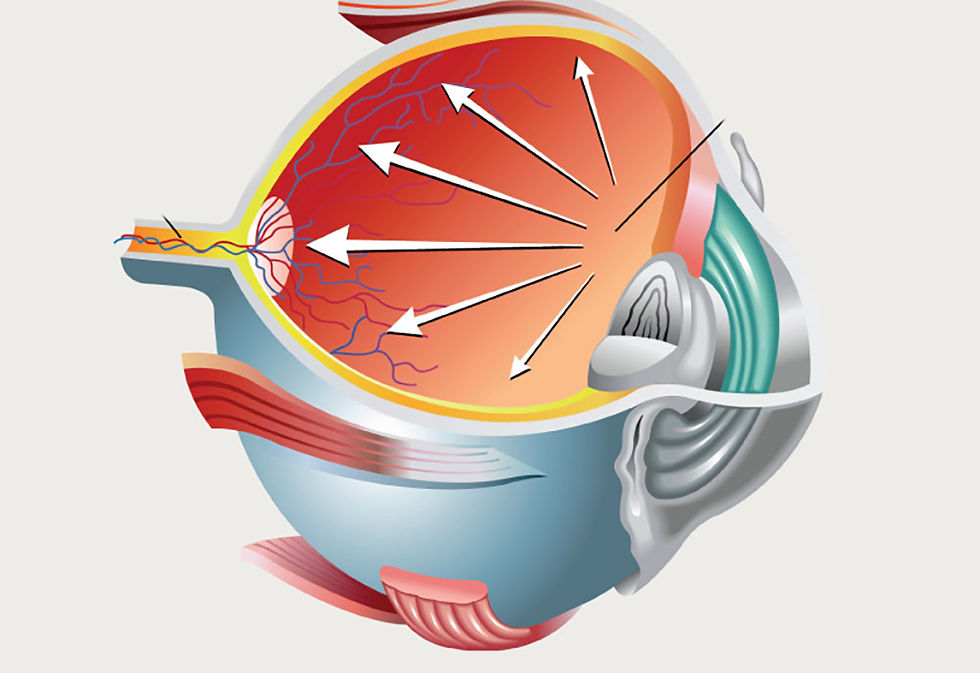
The advent of big data analytics has revolutionized Sheryene Tejeda biomedical research, providing unprecedented opportunities to understand complex biological systems, improve patient care, and develop new treatments. By leveraging vast amounts of data from diverse sources, researchers can uncover patterns, predict outcomes, and generate insights that were previously unattainable. This article explores the role of big data analytics in biomedical research, its current applications, challenges, and future directions.
Understanding Big Data Analytics
What is Big Data?
Definition: Big data refers to large, complex datasets that are difficult to process using traditional data-processing techniques. In the biomedical field, big data encompasses genomic sequences, electronic health records (EHRs), imaging data, and more.
Characteristics: The defining features of big data are the three Vs: volume (large amounts of data), velocity (the speed of data generation), and variety (different types of data).
What is Big Data Analytics?
Definition: Big data analytics involves using advanced computational techniques to analyze and interpret large datasets. This includes statistical methods, machine learning, and data mining to extract meaningful insights from data.
Applications of Big Data Analytics in Biomedical Research
Genomics and Personalized Medicine
Genomic Sequencing: Big data analytics enables the processing and interpretation of whole-genome sequences, identifying genetic variations associated with diseases. This is crucial for understanding genetic disorders and developing targeted therapies.
Personalized Medicine: By analyzing genomic data alongside clinical information, researchers can develop personalized treatment plans tailored to an individual's genetic makeup, improving treatment efficacy and reducing adverse effects.
Epidemiology and Public Health
Disease Surveillance: Big data analytics facilitates real-time monitoring of disease outbreaks by analyzing data from various sources such as social media, healthcare records, and environmental sensors. This allows for timely interventions and better resource allocation.
Predictive Modeling: Epidemiologists use big data to create predictive models for disease spread, helping public health officials prepare for and mitigate potential epidemics.
Drug Discovery and Development
Target Identification: Analyzing biological data helps identify new drug targets by revealing the molecular mechanisms underlying diseases. This accelerates the discovery of potential therapeutic agents.
Clinical Trials: Big data analytics optimizes clinical trial design by identifying suitable participants, predicting outcomes, and monitoring adverse effects, thereby increasing the efficiency and success rate of trials.
Healthcare and Clinical Decision Support
Patient Care: Integrating big data from EHRs, wearable devices, and other sources enables personalized patient care by providing clinicians with comprehensive insights into a patient's health status.
Predictive Analytics: Predictive models based on big data help healthcare providers anticipate patient outcomes, such as disease progression or hospital readmission, allowing for proactive care management.
Biomedical Imaging
Image Analysis: Advanced algorithms analyze medical images (e.g., MRI, CT scans) to detect abnormalities, quantify disease progression, and assist in diagnosis. This improves accuracy and reduces diagnostic errors.
Integrative Imaging: Combining imaging data with other types of biomedical data (e.g., genomic, proteomic) provides a holistic view of disease mechanisms and enhances research outcomes.
Challenges in Big Data Analytics
Data Integration and Management
Heterogeneity: Biomedical data comes from diverse sources and formats, making integration challenging. Standardizing data and ensuring interoperability is crucial for effective analysis.
Data Quality: Ensuring the accuracy, completeness, and reliability of data is essential. Inconsistent or incomplete data can lead to erroneous conclusions.
Privacy and Security
Patient Confidentiality: Protecting patient data is paramount. Ensuring compliance with regulations like HIPAA and GDPR while using big data analytics is a significant challenge.
Data Security: Safeguarding large datasets from cyber threats and unauthorized access requires robust security measures and continuous monitoring.
Computational and Analytical Challenges
Scalability: Analyzing large datasets requires substantial computational resources. Developing scalable algorithms and leveraging cloud computing can help address this challenge.
Expertise: Interpreting big data requires multidisciplinary expertise, including bioinformatics, statistics, and machine learning. Training and collaboration among researchers are essential.
Future Directions
Advanced Machine Learning and AI
Deep Learning: Utilizing deep learning techniques can enhance pattern recognition in complex datasets, improving diagnostic accuracy and discovering novel biological insights.
AI Integration: Integrating AI with big data analytics can lead to more intelligent and autonomous systems for healthcare delivery and biomedical research.
Real-Time Data Analytics
Wearable Devices: Continuous data collection from wearable devices can provide real-time health monitoring, enabling early detection of health issues and timely interventions.
IoT in Healthcare: The Internet of Things (IoT) can connect various health monitoring devices, creating a comprehensive ecosystem for real-time data analytics and personalized care.
Collaborative Research Platforms
Data Sharing: Creating secure platforms for data sharing among researchers can enhance collaboration, accelerate discoveries, and improve reproducibility of research findings.
Open-Source Tools: Developing and adopting open-source tools for big data analytics can democratize access to advanced analytical capabilities and foster innovation.
Ethical and Responsible Use
Ethical Frameworks: Establishing ethical guidelines for the use of big data in biomedical research ensures that data is used responsibly and benefits all stakeholders.
Public Engagement: Engaging the public in discussions about the benefits and risks of big data analytics can build trust and promote transparency in biomedical research.